View-agnostic Image Rendering
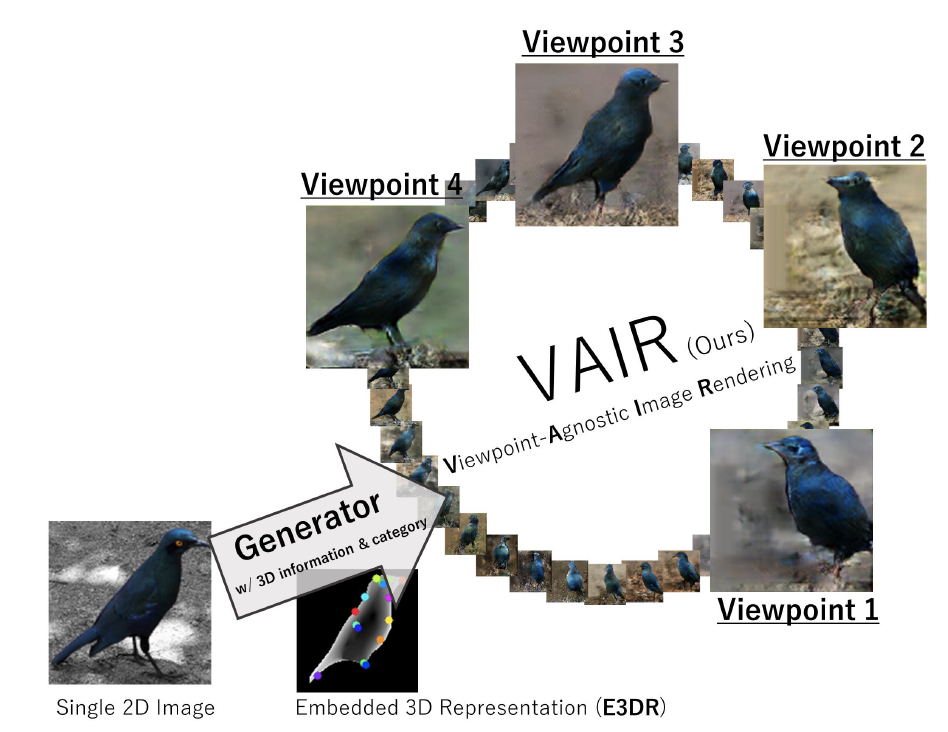
-
We generate novel-view images.
Rendering an any-viewpoint image is extremely difficult for Generative Adversarial Networks. This is because conventional GANs do not understand 3D information underlying a given viewpoint image such as an object shape and relationship between viewpoint and objects in 3D space. In this paper, we present how to perform a Viewpoint-Agnostic Image Rendering (VAIR), equipping a conditional GAN with a mechanism to reconstruct 3D information of the input view. VAIR realizes any-viewpoint image generation by manipulating a viewpoint in 3D space where the reconstructed instance shape is arranged. In addition, we convert the reconstructed 3D shape into a 2D representation for image-based conditional GAN, while preserving detail 3D information. The representation consists of a depth image and 2D semantic keypoint images, which are obtained by rendering the shape from a viewpoint. In the experiment, we evaluate using a CUB-200-2011 dataset, which contains few-samples biased a viewpoint such that covers only part of the target appearance. As a result, our VAIR clearly renders an any-viewpoint image.
-
Post destination
WACV 2021
-
Member
Hiroaki Aizawa (Gifu Univ.), Hirokatsu Kataoka (AIST), Yutaka Satoh (AIST), Kunihito Kato (Gifu Univ.)
Related Links